Dr. Ross Gore
Research Associate Professor
757 638 7002 | rgore@odu.edu
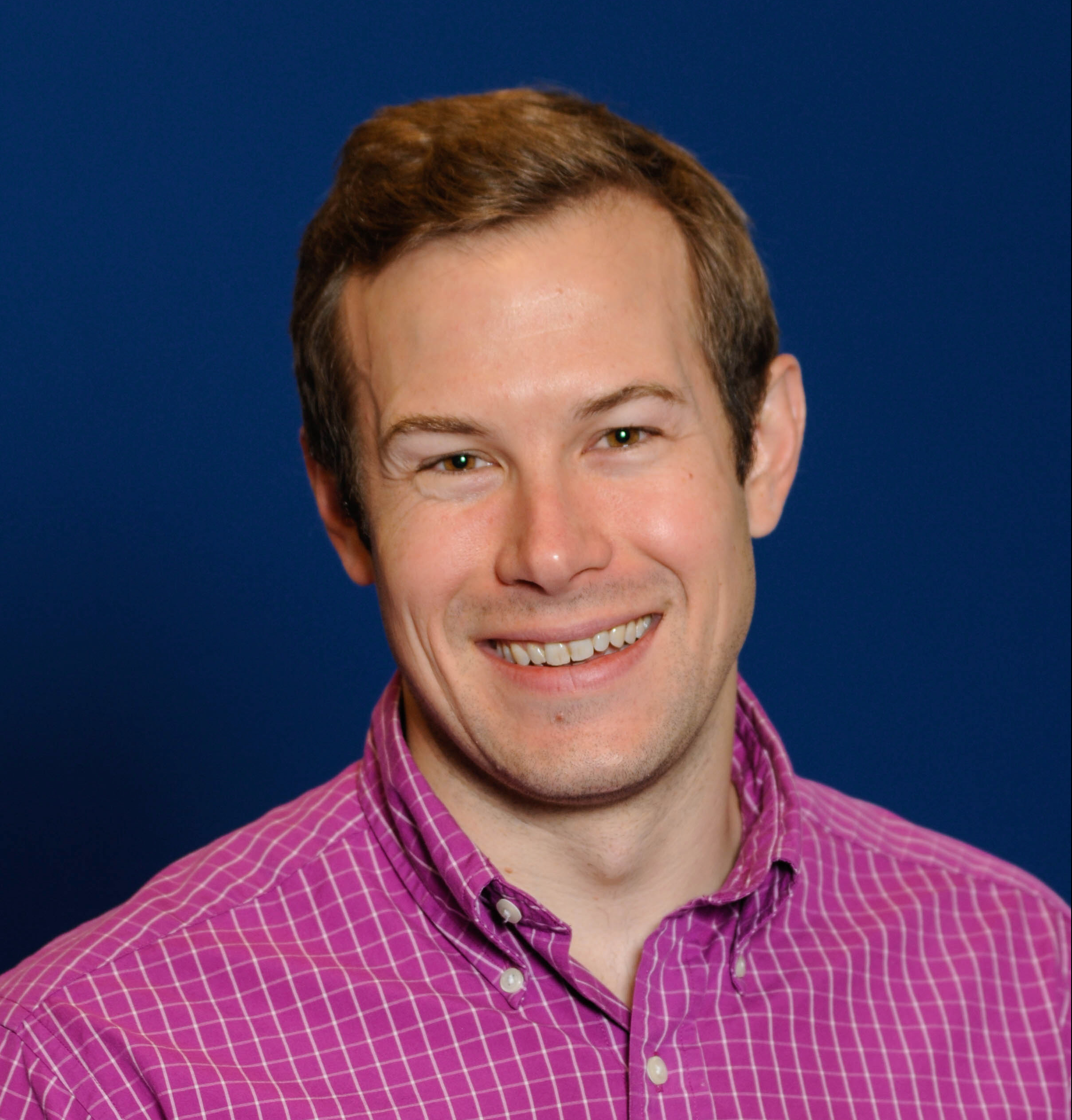
RESEARCH FIELD OF EXPERTISE & RESEARCH INTERESTS
Data Science, Predictive Analytics, Computer Science, Simulation Validation, Software Debugging
SHORT BIOGRAPHY
Ross Gore, Ph.D., is a Research Associate Professor at the Virginia Modeling, Analysis and Simulation Center (VMASC) at Old Dominion University (ODU). He holds a Doctorate of Philosophy (Ph.D.) and a Master's degree in Computer Science from the University of Virginia (UVA) and a Bachelor's degree in Computer Science from the University of Richmond (UR). His current work focuses on data science and predictive analytics.
Dr. Gore is passionate about extracting useful information from new and diverse data sources to help inform decision making. The domains of the decisions can vary from public health and city planning to risk assessments related to cyber and terrorist attacks. Typical research engagements involve in-person dialogue with stakeholders to understand the problem at hand, followed by an exploration of problem specific data including existing data that has been collected, publicly available data and the design of new data collection methods. Analytical insight is then produced iteratively through a series of rapid prototypes that enable the stakeholders to provide actionable feedback.
Recent research efforts include: (1) leveraging mobile phone data to inform public-health decisions, (2) identifying measures from Twitter content that explain the variance in the obesity rate across the United States, and (3) customizing cyber vulnerability assessments to provide a user-specific prioritized list of remedial action items.
EDUCATION
Ph.D. in Computer Science, 2012
University of Virginia (UVA), Charlottesville, VA
M.S. in Computer Science, 2007
University of Virginia (UVA), Charlottesville, VA
B.S. in Computer Science, 2003
University of Richmond (UR), Richmond, VA
PROFESSIONAL EXPERIENCE
VMASC, Research Associate Professor
1030 University Boulevard; Suffolk, VA
2014 – Present
Gettysburg College, Computer Science Department, Visiting Assistant Professor
300 N Washington Street; Gettysburg, PA
2013 - 2014
VMASC, Post-Doctoral Research Associate
1030 University Boulevard; Suffolk, VA
2012 - 2013
VIRGINIA COUNTY COVID-19 DAILY CASE TOTAL FORECASTER
The research platform allows the public to explore, at a very local level, how the number of reported COVID-19 cases is increasing or decreasing in different areas of the state, based on different models. The forecasting models show that some communities, such as Norfolk, have had more success leveling off the reporting of new cases than other localities. The platform also includes age ranges and hospitalization outcomes for the forecasted cases as well as links to a plethora of other COVID-19 modeling resources, and more.
MODELING RELIGION IN NORWAY (MODRN)
The goal of the MODRN project is to create cutting edge computer simulations of religious and social conflict in Norway, using modeling systems that enable "virtual" social experimentation by integrating empirically validated theories in the scientific study of religion (and secularization) within complex "causal architectures." These simulations will be calibrated using massive Norwegian datasets in dialogue with national and international experts in the fields of computer modeling, religious and secular diversification, and Norwegian public-policy. MODRN will produce new tools for evaluating hypotheses about - and policies for - religious and socio-political change in Norway, Northern Europe and beyond.
A MODEL-BASED FRAMEWORK FOR QUANTIFYING INFORMATION RISK RELATED TO CYBER VULNERABILITIES
This work is a collaboration with G2 Ops Inc. It provides a client specific cyber vulnerability assessment. Using existing products and frameworks we create a prioritized list of action items for Chief Security Officers (CSOs) to address based on the business values of their companies and the location of their assets. The technical approach conducts a vulnerability scan of the network with a COTS product. Next, it integrates client supplied data about business values and assets of each scanned IP address with the scan data. Finally we calculate the influence each IP address has on all others based on network topology. Visualize the client specific cyber vulnerability analysis.
YOU ARE WHAT YOU TWEET: CONNECTING THE GEOGRAPHIC VARIATION IN AMERICA'S OBESITY RATE TO TWITTER CONTENT
The research reflects a detailed investigation of the relationship among the obesity rate of urban areas and expressions of happiness, diet and physical activity on social media. We do so by analyzing a massive, geo-tagged data set comprising over 200 million words generated over the course of 2012 and 2013 on the social network service Twitter. Among many results, we show that areas with lower obesity rates: (1) have happier tweets and frequently discuss (2) food, particularly fruits and vegetables, and (3) physical activities of any intensity. Additionally, we provide evidence that each of these results offer different and unique insight into the variation of the obesity rate in urban areas within the United States. Our work shows how the contents of social media may potentially be used to estimate real-time, population-scale measures of factors related to obesity.
BIG DATA AS A PUBLIC HEALTH DECISION ENABLER
Our work facilitates health care-decision marking using mobile cell phone data from more than 300,000 Senegal citizens. From this data we identified: (1) the population density across the 14 regions of the country and (2) risk zones where citizens who suffered from either strokes or heart attacks (Myocardial infractions) would be unable to reach a hospital to receive treatment in 90 minutes or less. In combination these two results informed decision makers about where to establish future Senegal hospitals to minimize stroke and heart attack risk zones. The result will reduce the cost and accelerate the delivery of health-care infrastructure across Senegal. This research received an award from the Data for Development Senegal (D4D) sponsored by The Bill & Melinda Gates Foundation.
JOURNAL PUBLICATIONS
Gore, Ross Joseph, Saikou Diallo, and Jose Padilla. "You are what you tweet: connecting the geographic variation in america’s obesity rate to Twitter content." PloS one 10.9 (2015). https://journals.plos.org/plosone/article?id=10.1371/journal.pone.0133505
Gore, Ross, et al. "Forecasting changes in religiosity and existential security with an agent-based model." Journal of Artificial Societies and Social Simulation 21.1 (2018). http://jasss.soc.surrey.ac.uk/21/1/4.html
Gore, Ross J., Christopher J. Lynch, and Hamdi Kavak. "Applying statistical debugging for enhanced trace validation of agent-based models." Simulation 93.4 (2017): 273-284.
Gore, Ross, et al. "Statistical debugging for simulations." ACM Transactions on Modeling and Computer Simulation (TOMACS) 25.3 (2015): 1-26.
Gore, Ross J., et al. "Augmenting bottom-up metamodels with predicates." Jasss: The Journal of Artificial Societies and Social Simulation 20.1 (2017). http://jasss.soc.surrey.ac.uk/20/1/4.html
PEER-REVIEWED CONFERENCE PAPERS
Gore, Ross, and Paul F. Reynolds. "Reducing confounding bias in predicate-level statistical debugging metrics." 2012 34th International Conference on Software Engineering (ICSE). IEEE, 2012.
Gore, Ross, and Paul F. Reynolds. "Applying causal inference to understand emergent behavior." 2008 Winter Simulation Conference. IEEE, 2008.
Gore, Ross, Paul F. Reynolds, and David Kamensky. "Statistical debugging with elastic predicates." 2011 26th IEEE/ACM International Conference on Automated Software Engineering (ASE 2011). IEEE, 2011.
CV/RESUME
Dr. Ross Gore
Research Associate Professor
757 638 7002 | rgore@odu.edu
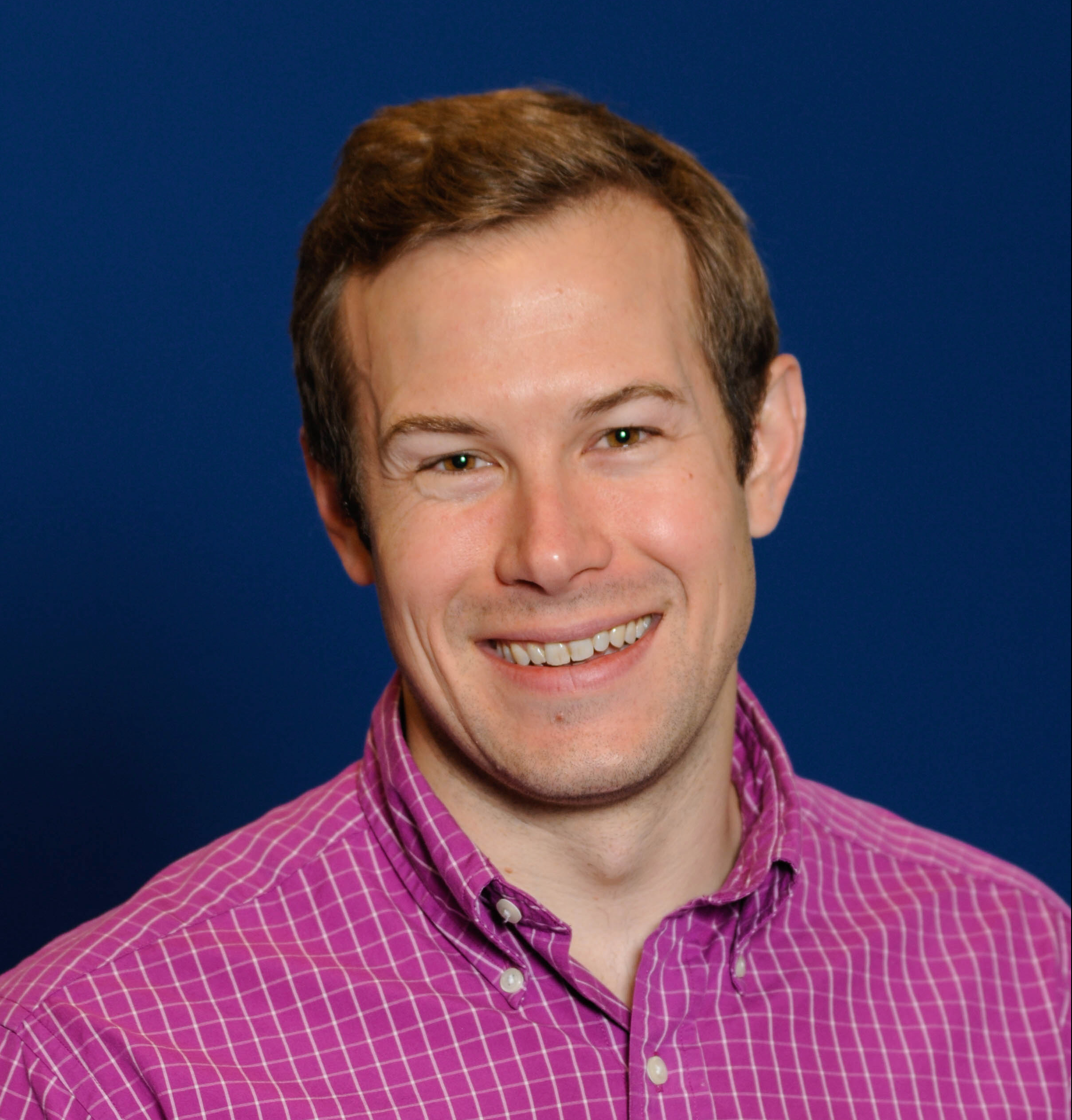
RESEARCH FIELD OF EXPERTISE & RESEARCH INTERESTS
Data Science, Predictive Analytics, Computer Science, Simulation Validation, Software Debugging
SHORT BIOGRAPHY
Ross Gore, Ph.D., is a Research Associate Professor at the Virginia Modeling, Analysis and Simulation Center (VMASC) at Old Dominion University (ODU). He holds a Doctorate of Philosophy (Ph.D.) and a Master's degree in Computer Science from the University of Virginia (UVA) and a Bachelor's degree in Computer Science from the University of Richmond (UR). His current work focuses on data science and predictive analytics.
Dr. Gore is passionate about extracting useful information from new and diverse data sources to help inform decision making. The domains of the decisions can vary from public health and city planning to risk assessments related to cyber and terrorist attacks. Typical research engagements involve in-person dialogue with stakeholders to understand the problem at hand, followed by an exploration of problem specific data including existing data that has been collected, publicly available data and the design of new data collection methods. Analytical insight is then produced iteratively through a series of rapid prototypes that enable the stakeholders to provide actionable feedback.
Recent research efforts include: (1) leveraging mobile phone data to inform public-health decisions, (2) identifying measures from Twitter content that explain the variance in the obesity rate across the United States, and (3) customizing cyber vulnerability assessments to provide a user-specific prioritized list of remedial action items.
EDUCATION
Ph.D. in Computer Science, 2012
University of Virginia (UVA), Charlottesville, VA
M.S. in Computer Science, 2007
University of Virginia (UVA), Charlottesville, VA
B.S. in Computer Science, 2003
University of Richmond (UR), Richmond, VA
PROFESSIONAL EXPERIENCE
VMASC, Research Associate Professor
1030 University Boulevard; Suffolk, VA
2014 – Present
Gettysburg College, Computer Science Department, Visiting Assistant Professor
300 N Washington Street; Gettysburg, PA
2013 - 2014
VMASC, Post-Doctoral Research Associate
1030 University Boulevard; Suffolk, VA
2012 - 2013
VIRGINIA COUNTY COVID-19 DAILY CASE TOTAL FORECASTER
The research platform allows the public to explore, at a very local level, how the number of reported COVID-19 cases is increasing or decreasing in different areas of the state, based on different models. The forecasting models show that some communities, such as Norfolk, have had more success leveling off the reporting of new cases than other localities. The platform also includes age ranges and hospitalization outcomes for the forecasted cases as well as links to a plethora of other COVID-19 modeling resources, and more.
MODELING RELIGION IN NORWAY (MODRN)
The goal of the MODRN project is to create cutting edge computer simulations of religious and social conflict in Norway, using modeling systems that enable "virtual" social experimentation by integrating empirically validated theories in the scientific study of religion (and secularization) within complex "causal architectures." These simulations will be calibrated using massive Norwegian datasets in dialogue with national and international experts in the fields of computer modeling, religious and secular diversification, and Norwegian public-policy. MODRN will produce new tools for evaluating hypotheses about - and policies for - religious and socio-political change in Norway, Northern Europe and beyond.
A MODEL-BASED FRAMEWORK FOR QUANTIFYING INFORMATION RISK RELATED TO CYBER VULNERABILITIES
This work is a collaboration with G2 Ops Inc. It provides a client specific cyber vulnerability assessment. Using existing products and frameworks we create a prioritized list of action items for Chief Security Officers (CSOs) to address based on the business values of their companies and the location of their assets. The technical approach conducts a vulnerability scan of the network with a COTS product. Next, it integrates client supplied data about business values and assets of each scanned IP address with the scan data. Finally we calculate the influence each IP address has on all others based on network topology. Visualize the client specific cyber vulnerability analysis.
YOU ARE WHAT YOU TWEET: CONNECTING THE GEOGRAPHIC VARIATION IN AMERICA'S OBESITY RATE TO TWITTER CONTENT
The research reflects a detailed investigation of the relationship among the obesity rate of urban areas and expressions of happiness, diet and physical activity on social media. We do so by analyzing a massive, geo-tagged data set comprising over 200 million words generated over the course of 2012 and 2013 on the social network service Twitter. Among many results, we show that areas with lower obesity rates: (1) have happier tweets and frequently discuss (2) food, particularly fruits and vegetables, and (3) physical activities of any intensity. Additionally, we provide evidence that each of these results offer different and unique insight into the variation of the obesity rate in urban areas within the United States. Our work shows how the contents of social media may potentially be used to estimate real-time, population-scale measures of factors related to obesity.
BIG DATA AS A PUBLIC HEALTH DECISION ENABLER
Our work facilitates health care-decision marking using mobile cell phone data from more than 300,000 Senegal citizens. From this data we identified: (1) the population density across the 14 regions of the country and (2) risk zones where citizens who suffered from either strokes or heart attacks (Myocardial infractions) would be unable to reach a hospital to receive treatment in 90 minutes or less. In combination these two results informed decision makers about where to establish future Senegal hospitals to minimize stroke and heart attack risk zones. The result will reduce the cost and accelerate the delivery of health-care infrastructure across Senegal. This research received an award from the Data for Development Senegal (D4D) sponsored by The Bill & Melinda Gates Foundation.
JOURNAL PUBLICATIONS
Gore, Ross Joseph, Saikou Diallo, and Jose Padilla. "You are what you tweet: connecting the geographic variation in america’s obesity rate to Twitter content." PloS one 10.9 (2015). https://journals.plos.org/plosone/article?id=10.1371/journal.pone.0133505
Gore, Ross, et al. "Forecasting changes in religiosity and existential security with an agent-based model." Journal of Artificial Societies and Social Simulation 21.1 (2018). http://jasss.soc.surrey.ac.uk/21/1/4.html
Gore, Ross J., Christopher J. Lynch, and Hamdi Kavak. "Applying statistical debugging for enhanced trace validation of agent-based models." Simulation 93.4 (2017): 273-284.
Gore, Ross, et al. "Statistical debugging for simulations." ACM Transactions on Modeling and Computer Simulation (TOMACS) 25.3 (2015): 1-26.
Gore, Ross J., et al. "Augmenting bottom-up metamodels with predicates." Jasss: The Journal of Artificial Societies and Social Simulation 20.1 (2017). http://jasss.soc.surrey.ac.uk/20/1/4.html
PEER-REVIEWED CONFERENCE PAPERS
Gore, Ross, and Paul F. Reynolds. "Reducing confounding bias in predicate-level statistical debugging metrics." 2012 34th International Conference on Software Engineering (ICSE). IEEE, 2012.
Gore, Ross, and Paul F. Reynolds. "Applying causal inference to understand emergent behavior." 2008 Winter Simulation Conference. IEEE, 2008.
Gore, Ross, Paul F. Reynolds, and David Kamensky. "Statistical debugging with elastic predicates." 2011 26th IEEE/ACM International Conference on Automated Software Engineering (ASE 2011). IEEE, 2011.
CV/RESUME